One-Sentence Project Summary
This summer, Margaret and Sagunya worked with their mentor, Dr. Eric Deutsch of the Moritz Lab at the renowned Institute for Systems Biology (ISB) to launch and document a second build of the annotated proteome of Arabidopsis thaliana on ISB’s PeptideAtlas website.
In-Depth Project Description
Project Background:
Quoting from the PeptideAtlas website, “The long term goal of the PeptideAtlas project is full annotation of eukaryotic genomes through a thorough validation of expressed proteins.”
In the fall of 2021, members of the Moritz Lab at the Institute for Systems Biology (ISB), including Tami Leppert, Zhi Sun, and Luis Mendoza, partnered with researchers Klaas van Wijk and Qi Sun from Cornell University to create and publish a new build for the proteome of the model plant, Arabidopsis thaliana.
Like the fruit fly and the mouse, Arabidopsis thaliana is frequently studied by researchers around the world. The Arabidopsis proteome has been especially useful for research surrounding gene functions and molecular pathways. Since its establishment as a recognized model plant system in the 1980s, Arabidopsis has been a crucial tool in advancing plant biology. Just last year, PeptideAtlas launched a first build of the Arabidopsis proteome. This build makes accessible valuable information like protein splice forms and post-translational modifications as well as contains detailed profiles of specific proteins.
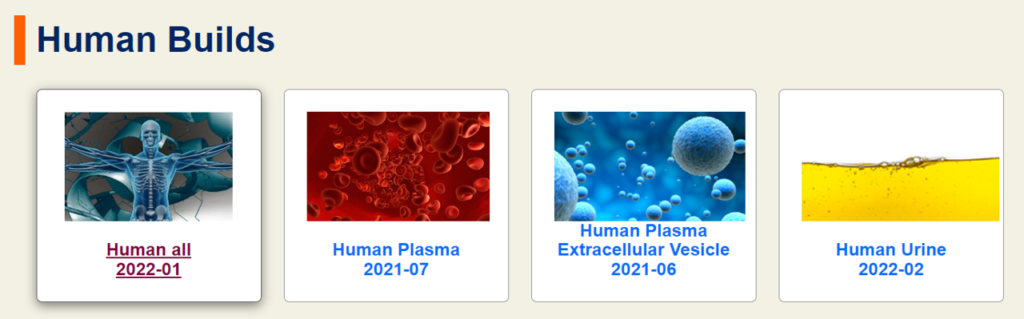
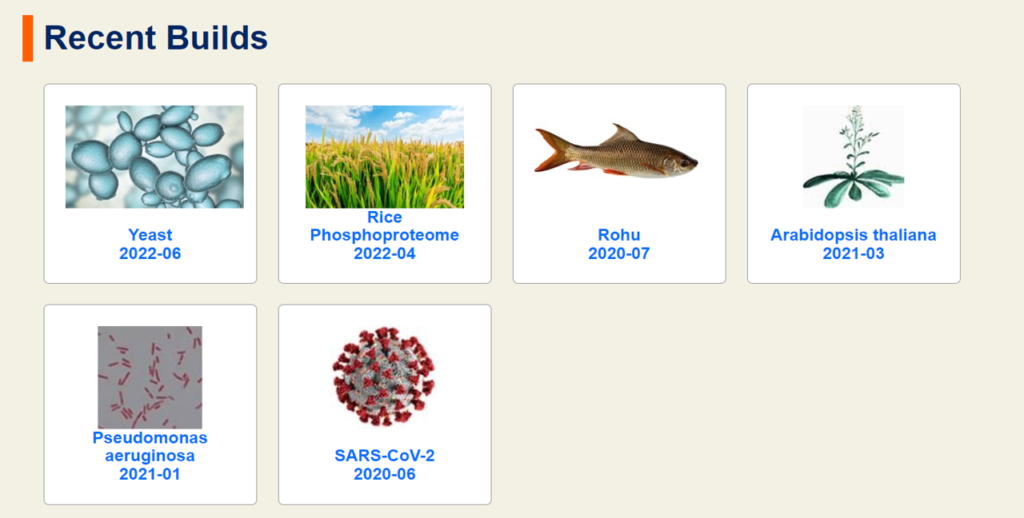
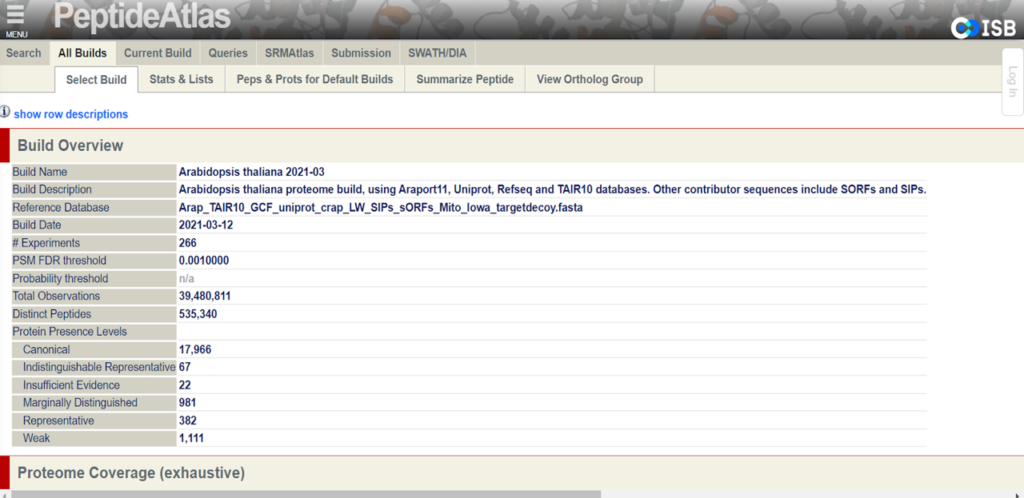
The goal of the second build which will be released in the fall of 2022 is to provide the proteomics community with a more robustly annotated version of the Arabidopsis proteome. Specifically, the second build will include information about previously undetected proteins and more detailed metadata of cited protein datasets. The new data that will be presented on PeptideAtlas primarily comes from the uniform processing of 217 million MS/MS raw spectra from 115 datasets from ProteomeXchange.
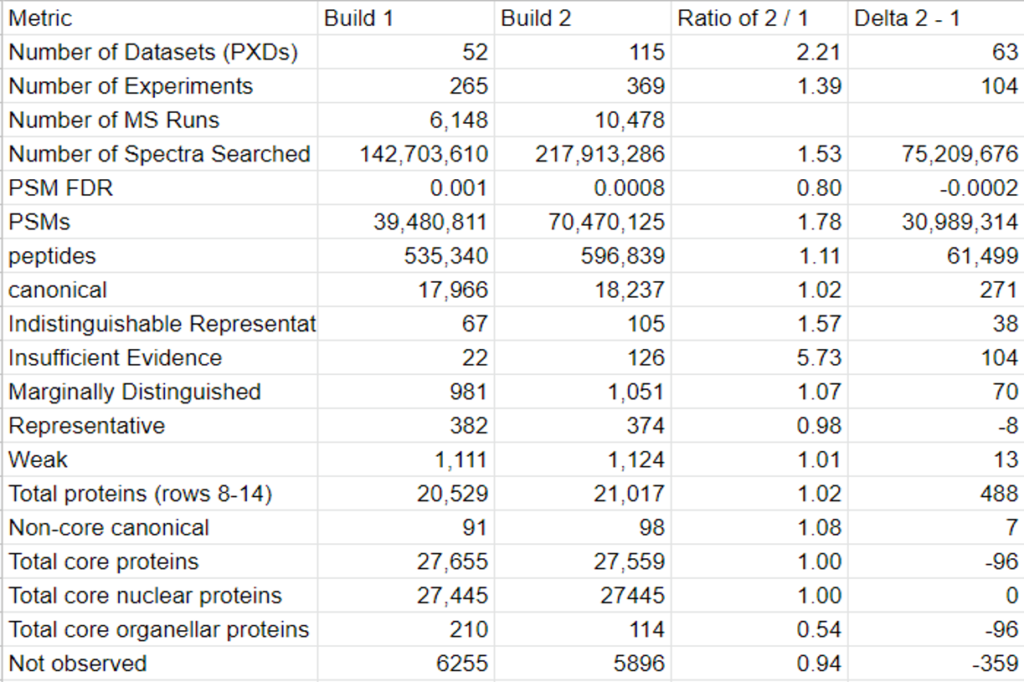
Samples of Our Contribution:
This year, the researchers of the PeptideAtlas project are planning on publishing three papers that document the creation of the second Arabidopsis build as well as the new insights they discovered in their research process. We, the high school interns of this project, mostly contribute by generating figures for the research papers that illustrate the research process. These figures can take the form of Excel tables or graphs. In the process of accomplishing these tasks, we also help extract, clean, and organize research data.

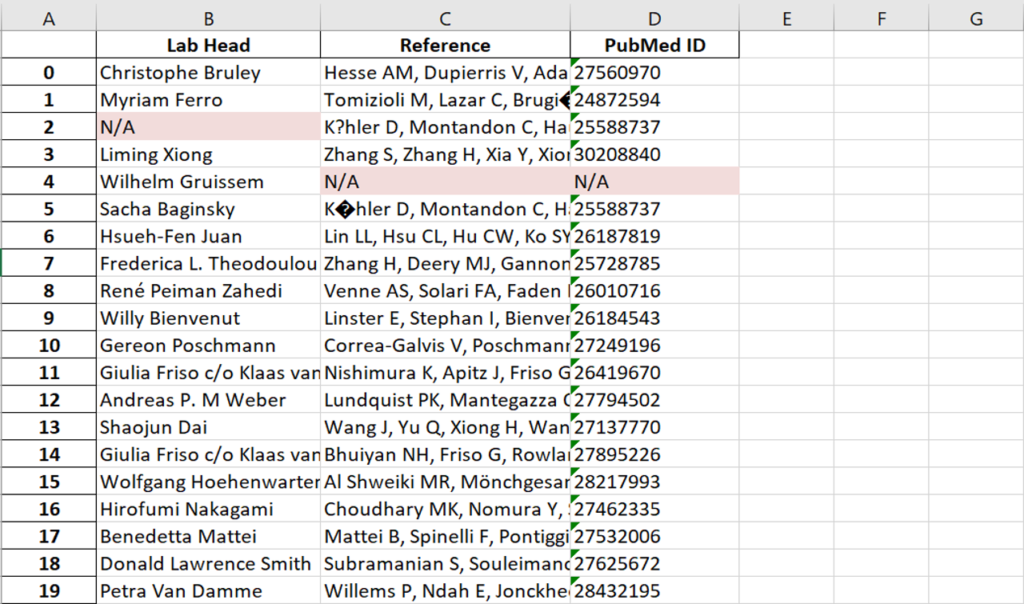
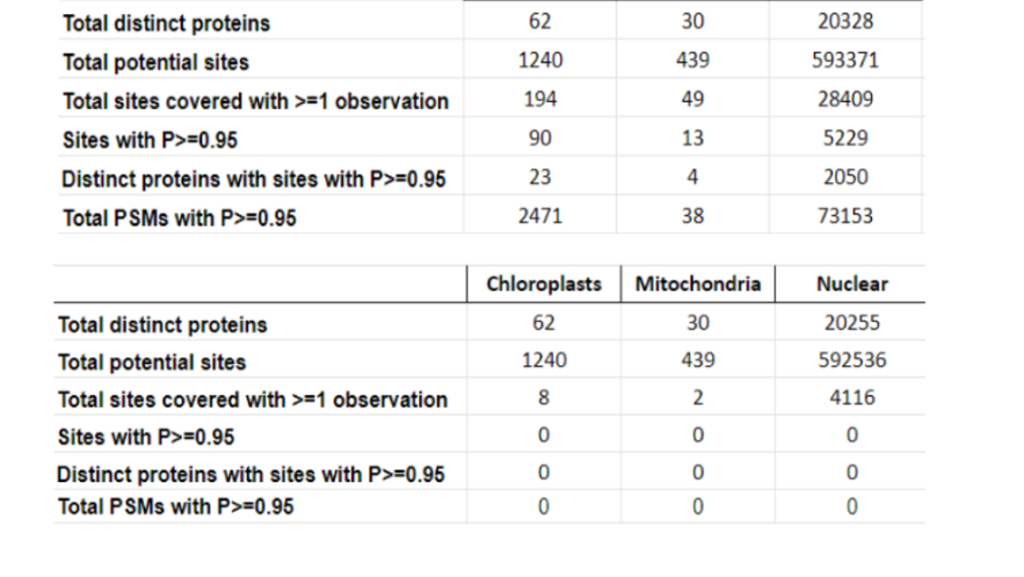
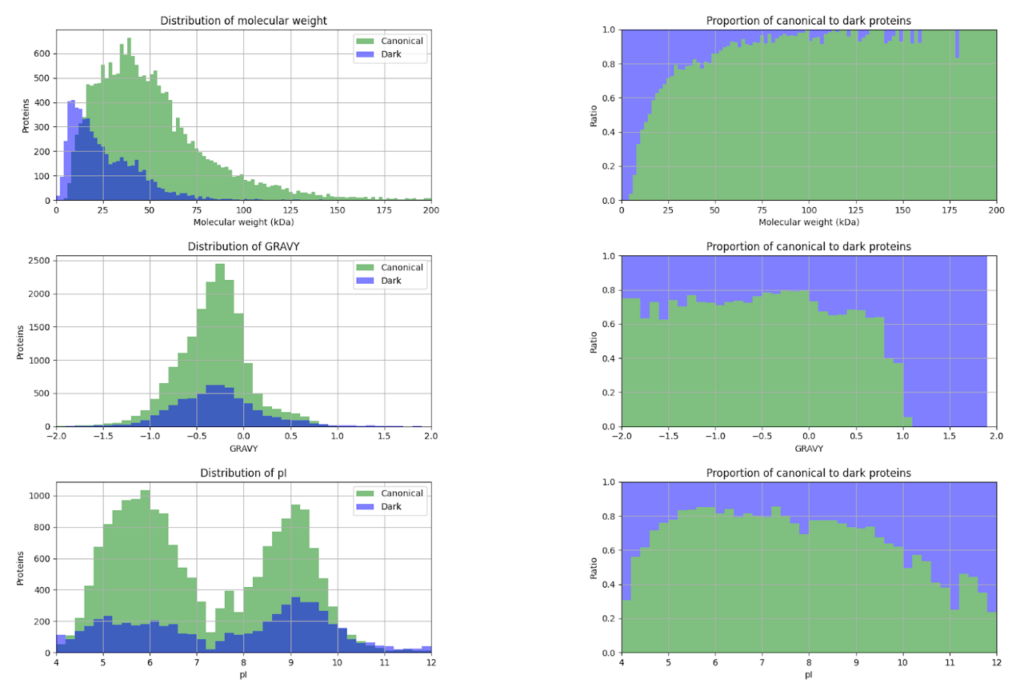
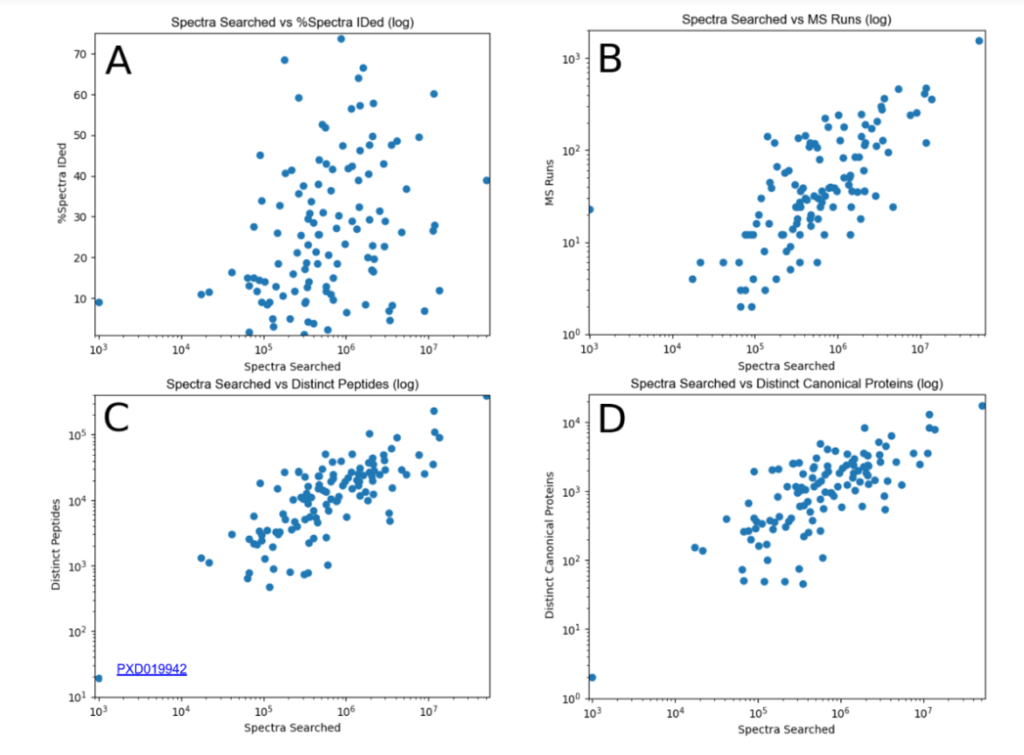
Acknowledgement
We would like to thank our mentor, Eric Deutsch for answering our never-ending well of questions and for helping us explore the complexities of his research with the greatest patience. Thank you to Claudia Ludwig, Miranda Johnson, Becky Howsmon, Jen Eklund, and of course, Nitin Baliga for guiding us through the internship experience and making this opportunity the most wonderful it could have been. Thank you to Jim Heath, Eric Deutsch, Lee Hood, Guangrong Qin, Dave Gibbs, and Anya Shukla for letting us interview you and generously sharing your experiences. Lastly, thank you to the people of ISB for being so friendly and welcoming to us. We are so, so grateful for all of you.